Cells in multicellular organisms share the same genome, but the differences in internal gene regulatory networks and external signaling from spatially neighboring cells in the surrounding microenvironment may cause dramatic variations in their morphology, gene expression, and function. Adjacent cells of different cell types interact through complex interactions to form tissue modules with specific functions. During the past few years, the development of spatial transcriptomics (ST) technologies has enabled the profiling of gene expression while retaining information on spatial location within a tissue. Therefore, the core task of ST data analysis is to effectively utilize this spatial information to reveal the spatial arrangement patterns of cells within complex tissues and their associated biological functions, which involves identifying spatially informed cell types and discovering tissue modules.
Currently, ST data analysis confronts two primary challenges. First, for spatially informed cell type identification, many studies were solely based on gene expression data, while the spatial information generated by ST methods has been used only rarely. Nevertheless, focused studies based on ST and related technologies are starting to accumulate evidence that cells that were originally considered as a homogeneous cell type can be further grouped into subtypes depending on their different locations in a tissue. Second, for the discovery of tissue modules, the current methods primarily rely on the coherent spatial expression patterns within regions of the tissue to discover tissue modules. However, from a functional perspective, tissue modules often consist of heterogeneous cell types with distinctive gene expression profiles that are spatially distributed in specific patterns. These methods do not fully leverage heterogeneous cell types' spatial organization within a tissue.
On May 31st, 2024, the research group led by Associate Professor Qiangfeng Cliff Zhang from the School of Life Sciences, Tsinghua University / Beijing Advanced Innovation Center for Structural Biology & Frontier Research Center for Biological Structure, Center for Synthetic and Systems Biology / Tsinghua-Peking Center for Life Sciences published a research paper titled “Tissue module discovery in single-cell resolution spatial transcriptomics data via cell-cell interaction-aware cell embedding” in the journal Cell Systems. In this study, they developed an artificial intelligence (AI) method named SPACE, based on a deep learning framework of graph auto-encoder, that can identify spatially informed cell types and discover tissue modules in ST data (Figure 1).
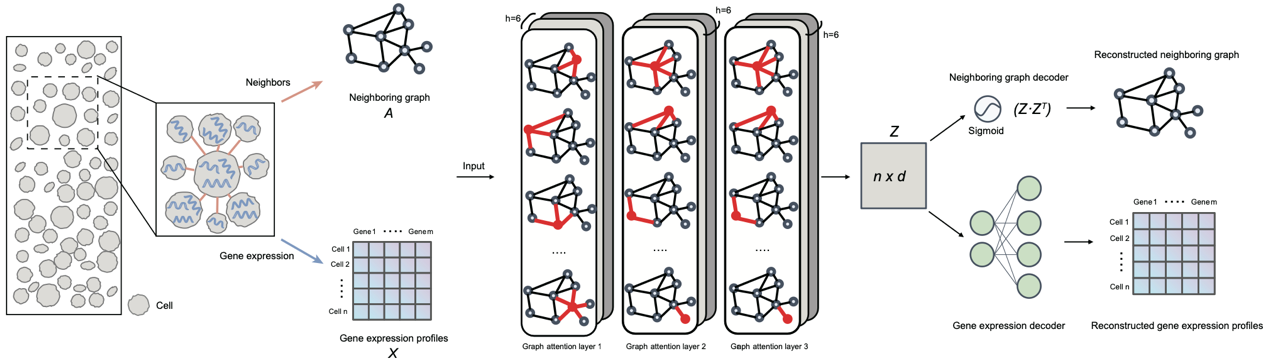
Figure 1. Overview of the SPACE method.
SPACE uses a unified graph auto-encoder framework to learn a low-dimensional representation of each analyte cell that reflects its gene expression profile and remembers the spatial organization of its neighboring interacting cells (therefore, this cell representation is referred to as cell-cell interaction-aware cell embedding). The learned cell representations can then be used for cell type identification and tissue module discovery using various clustering algorithms. SPACE distinguishes itself from currently available methods in two aspects. First, SPACE requires the reconstruction of both the gene expression profiles and the neighboring graph from the same low-dimensional cell representation (through two discrete decoders). Second, SPACE defines a perception field ratio α that determines the relative weight of the reconstruction loss of the gene expression profile and that of the neighboring graph. This adjustable ratio enables SPACE to shift its learning focus toward emphasizing (based on the specific research needs) either the gene expression profiles of each analyte cell or the organization of spatially neighboring cells. Thus, SPACE can identify spatially informed cell types or discover cell communities (CCs), a tissue module with discernible boundaries and a uniform spatial distribution of constituent cell types. When tested on various ST datasets, SPACE discovered CCs that share spatial patterns with manually annotated anatomical tissue structures and with previously described spatial domains; uniquely, the CCs are defined by their similar proximal cell-cell interactions rather than by coherent gene expression. These proximal cell-cell interaction networks can be used to constrain and refine cell-cell communication analyses by reducing the false discovery of ligand-receptor interactions that are unlikely to occur in space, thus improving the interpretation of the precise regulatory intercellular signaling events underlying these biological processes.
In summary, researchers have developed ST data analysis via interaction-aware cell embedding (SPACE), a deep-learning method for cell type identification and tissue module discovery from single-cell-resolution ST data. They envision that SPACE can be used in large-scale ST projects to understand how proximal cell-cell interactions contribute to emergent biological functions within cell communities.
Qiangfeng Cliff Zhang is the corresponding author of the paper, with Yuzhe Li, a Ph.D. graduate of class 2024 from the School of Life Sciences, Tsinghua University, and Jinsong Zhang, a postdoctoral fellow, serving as co-first authors. Professor Xin Gao, Director of the Computational Bioscience Research Center at King Abdullah University of Science and Technology and former AI scientist and Director of the Institute at BioMap, participated in the collaborative research.
The research was supported by the National Key Research and Development Project of China, the National Natural Science Foundation of China, the Beijing Advanced Innovation Center for Structural Biology, the Tsinghua-Peking Joint Center for Life Sciences, the Tsinghua University Computing Platform, the Shanghai Qi Zhi Institute, and the Office of Research Administration at King Abdullah University of Science and Technology.
Link to the paper: https://www.cell.com/cell-systems/fulltext/S2405-4712(24)00124-8
Editor: Li Han