Recently, the team led by Professor Shouliang Qi from the College of Medicine and Biological Information Engineering of NEU, in collaboration with the University of California, Los Angeles (UCLA), published a research paper titled "Spatial resolution enhancement using deep learning improves chest disease diagnosis based on thick slice CT" in npj Digital Medicine, a top global digital medicine journal (a sub-journal of Nature). Pengxin Yu, a 2023 Doctoral Student of NEU, and Haoyue Zhang from the National Institutes of Health are the co-first authors, and NEU is the first completion unit. Professor Shouliang Qi and Professor Corey Arnold from UCLA are the co-corresponding authors.
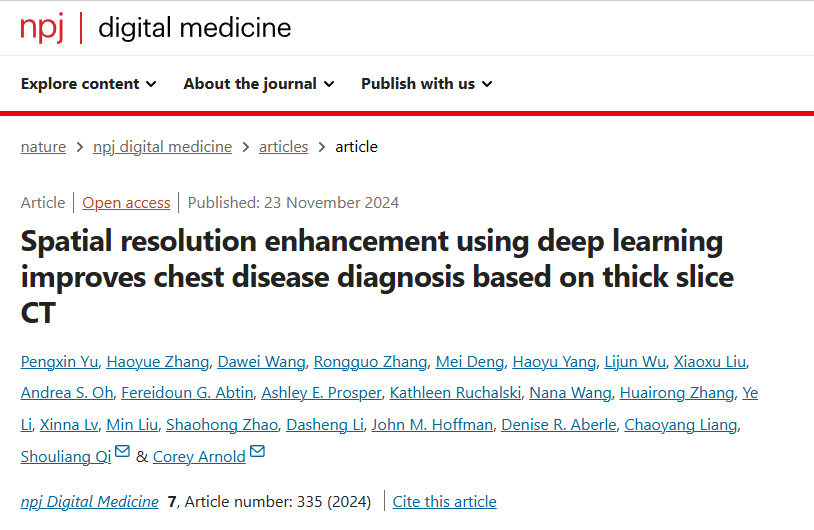
CT scan plays a crucial role in diagnosing chest diseases with image quality heavily affected by the spatial resolution. Due to the high cost of CT equipment and data storage, thick-slice CT scan is still prevalent in clinical practice, but its low spatial resolution may lead to misdiagnosis. To solve this problem, the collaborative team developed a deep learning-based medical image generation model through a multinational multi-center study, aiming to generate virtual thin-slice CT scan from thick-slice one.
To evaluate the quality of the virtual thin-slice CT scan, the research team invited 8 radiologists from home and abroad to conduct independent subjective evaluations. The results showed that the visual quality of the virtual thin-slice CT scan was comparable to that of the real thin-slice CT scan. In addition, 4 radiologists used the real thin-slice CT scan, the original thick-slice CT scan, and the virtual thin-slice CT scan for pneumonia diagnosis and lung nodule detection respectively. The study found that the virtual thin-slice CT scan significantly outperformed the original thick-slice CT scan in diagnostic performance and was comparable to the real thin-slice CT scan. Further experiments showed that the virtual thin-slice CT could also significantly improve performance when used with AI-assisted diagnostic software. Therefore, when thin-slice CT scan is unavailable, the virtual thin-slice CT scan generated by the proposed method can be an effective alternative, especially helpful for improving the healthcare situation in underdeveloped regions or countries.