为了加强我院青年学者与新加坡国立大学计算机系青年学者间的学术交流与合作,更好地推动我院计算机科学与技术学科的发展,同时加强与新加坡国立大学计算机学院之间的协同合作,华中科技大学计算机学院拟于2021年12月23日上午8:30-11:30在线上召开“2021华中科技大学计算机学院与新加坡国立大学计算机学院青年学者联合论坛暨‘TIME·青椒’沙龙第三期” (2021 Joint Symposium on Hot Topics On Computer System Research),本次活动由计算机学院青年教师联合会协办。
论坛旨在为计算机学科海内外青年才俊搭建学术交流平台和学术成果展示平台,通过专题报告、学术研讨和提问交流等形式,围绕计算机系统国际科学前沿热点研究领域展开研讨,促进海内外青年学者交流,增强计算机学科研究领域的国际交流与合作,助力青年教师发展。
论坛将以线上学术报告形式展开,ZOOM会议ID:917 0465 2865密码:163558
一、议程安排
2021华中科技大学计算机学院青年学者论坛议程安排 |
主办方与特邀嘉宾致辞 |
8:30-8:35 |
华中科技大学计算机学院副院长 石宣化 致辞 |
新加坡国立大学计算机学院副院长 何丙胜 致辞 |
会议报告 |
时间 |
报告题目 |
报告人 |
单位 |
主持人 |
08:35-09:00 |
Parallel Graph Processing Systems on Heterogeneous Architectures |
Bingsheng He |
新加坡国立大学计算机学院 |
胡燏翀 |
09:00-09:25 |
Exploiting Combined Locality for Wide- |
Yuchong Hu |
华中科技大学计算机学院 |
09:25-09:50 |
Colossal-AI: A Unified Deep Learning System For Large-Scale Parallel Training |
Yang You |
新加坡国立大学计算机学院 |
09:50-10:00 |
中场合影和休息 |
10:00-10:25 |
Co-Designing Distributed Systems with Programmable Networking Hardware |
Jialin Li |
新加坡国立大学计算机学院 |
胡燏翀 |
10:25-10:45 |
LogECMem: Coupling Erasure-Coded In-Memory Key-Value Stores with Parity Logging |
Liangfeng Cheng |
华中科技大学计算机学院 |
10:45-11:05 |
Accelerating Graph Convolutional Networks Using Crossbar-based Processing-In-Memory Architectures |
Yu Huang |
华中科技大学计算机学院 |
11:05-11:25 |
Efficient Algorithms for Set/String Similarity Search |
Zhong Yang |
华中科技大学计算机学院 |
11:25-11:30 |
华中科技大学计算机学院副院长 石宣化 结束语 |
二、论坛报告专家和主题简介:
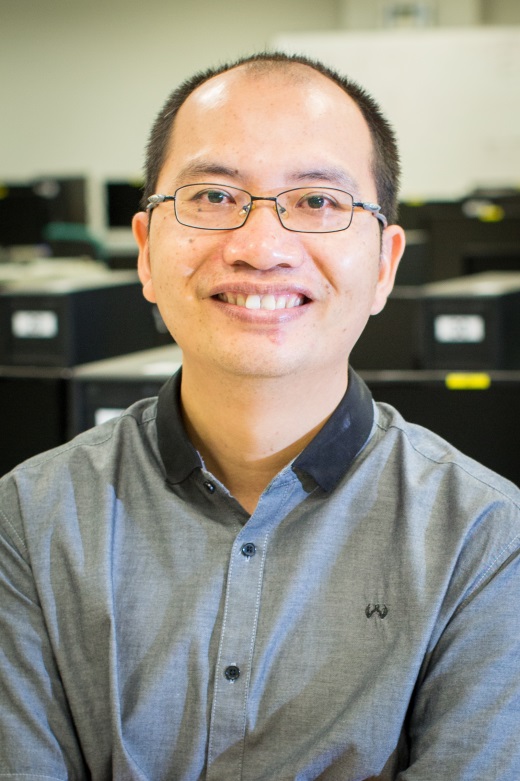
Bingsheng He
National University of Singapore,Dean’s Chair Associate Professor
Personal profile:Dr. Bingsheng He is currently a Dean’s Chair Associate Professor and Vice-Dean (Research) at School of Computing, National University of Singapore. Before that, he was a faculty member in Nanyang Technological University, Singapore (2010-2016), and held a research position in the System Research group of Microsoft Research Asia (2008-2010), where his major research was building high performance cloud computing systems for Microsoft. He got the Bachelor degree in Shanghai Jiao Tong University (1999-2003), and the Ph.D. degree in Hong Kong University of Science & Technology (2003-2008). His current research interests include cloud computing, database systems and high performance computing. His papers are published in prestigious international journals (such as ACM TODS and IEEE TKDE/TPDS/TC) and proceedings (such as ACM SIGMOD, VLDB/PVLDB, ACM/IEEE SuperComputing, ACM HPDC, and ACM SoCC). He has been awarded with the IBM Ph.D. fellowship (2008), NVIDIA Academic Partnership (2011), Adaptive Compute Research Cluster from Xilinx (2020) and ACM distinguished member (class 2020). Since 2010, he has (co-)chaired a number of international conferences and workshops, including IEEE CloudCom 2014/2015, BigData Congress 2018 and ICDCS 2020. He has served in editor board of international journals, including IEEE Transactions on Cloud Computing (IEEE TCC), IEEE Transactions on Parallel and Distributed Systems (IEEE TPDS), IEEE Transactions on Knowledge and Data Engineering (TKDE), Springer Journal of Distributed and Parallel Databases (DAPD) and ACM Computing Surveys (CSUR). He is an ACM Distinguished member (class of 2020).
Topic:Parallel Graph Processing Systems on Heterogeneous Architectures
Abstract:
Graphs are de facto data structures for many data processing applications, and their volume is ever growing. Many graph processing tasks are computation intensive and/or memory intensive. Therefore, we have witnessed a significant amount of effort in accelerating graph processing tasks with heterogeneous architectures like GPUs, FPGAs and even ASIC. In this talk, we will first review the literatures of large graph processing systems on heterogeneous architectures. Next, we present our research efforts, and demonstrate the significant performance impact of hardware-software co-design on designing high performance graph computation systems and applications. Finally, we outline the research agenda on challenges and opportunities in the system and application development of future graph processing. More details about our research can be found athttp://www.comp.nus.edu.sg/~hebs/.
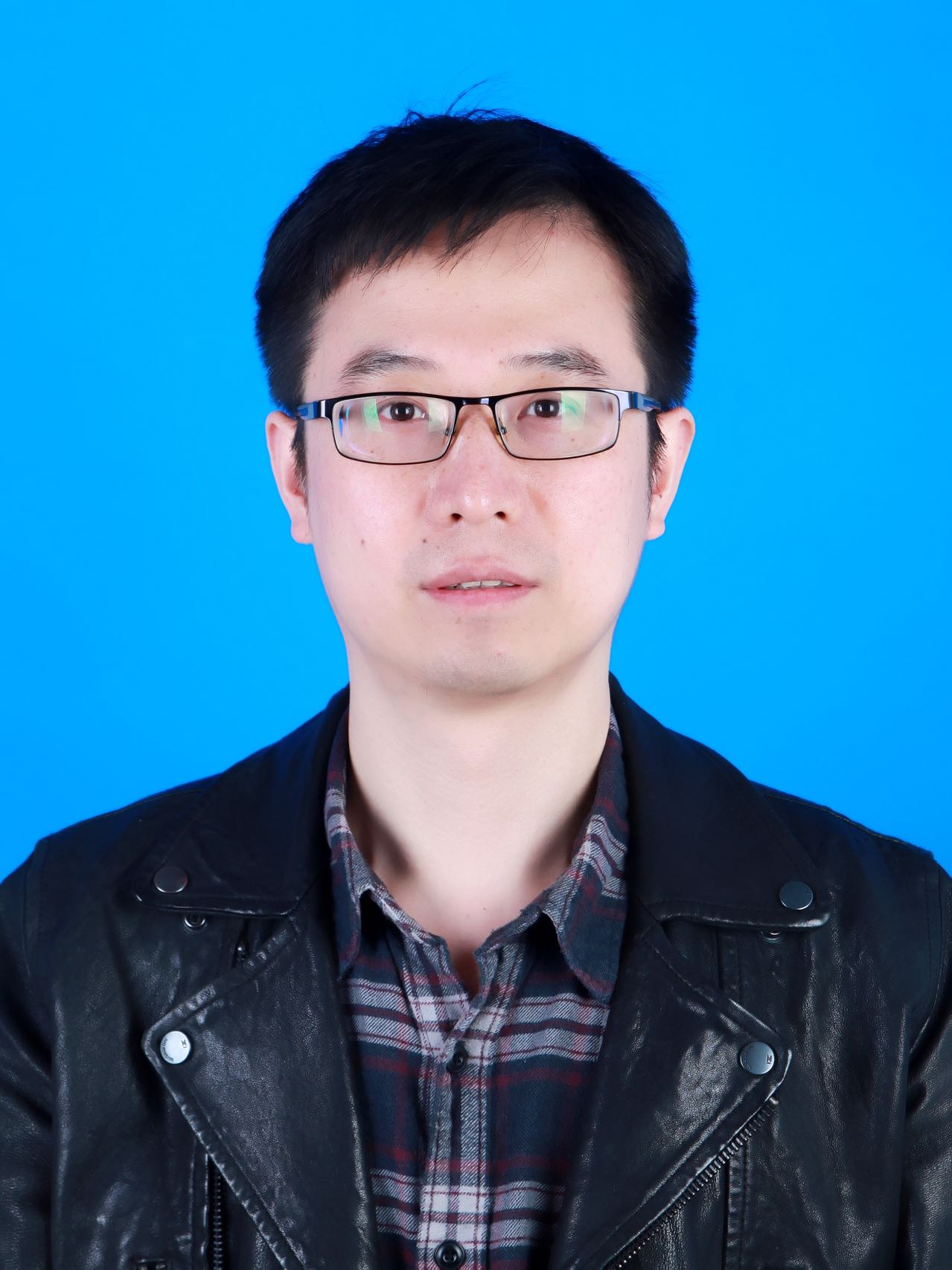
Yuchong Hu
Huazhong University of Science and Technology,Professor of the School of Computer Science and Technology
Personal profile:Yuchong Hu is a Professor of the School of Computer Science and Technology at the Huazhong University of Science and Technology (HUST). His research mainly focuses on designing and implementing storage systems with both high performance and dependability based on erasure coding and deduplication techniques, where the storage systems include cloud storage, big data storage, in-memory KV stores, backup storage, blockchain storage, etc.
Topic:Exploiting Combined Locality for Wide-Stripe Erasure Coding in Distributed Storage
Abstract(Optional):
Erasure coding is a low-cost redundancy mechanism for distributed storage systems by storing stripes of data and parity chunks. Wide stripes are recently proposed to suppress the fraction of parity chunks in a stripe to achieve extreme storage savings. However, wide stripes aggravate the repair penalty, while existing repair-efficient approaches for erasure coding cannot effectively address wide stripes. In this paper, we propose combined locality, the first mechanism that systematically addresses the wide-stripe repair problem via the combination of both parity locality and topology locality. We further augment combined locality with efficient encoding and update schemes. Experiments on Amazon EC2 show that combined locality reduces the single-chunk repair time by up to 90.5% compared to locality-based state-of-the-arts, with only a redundancy of as low as 1.063×.
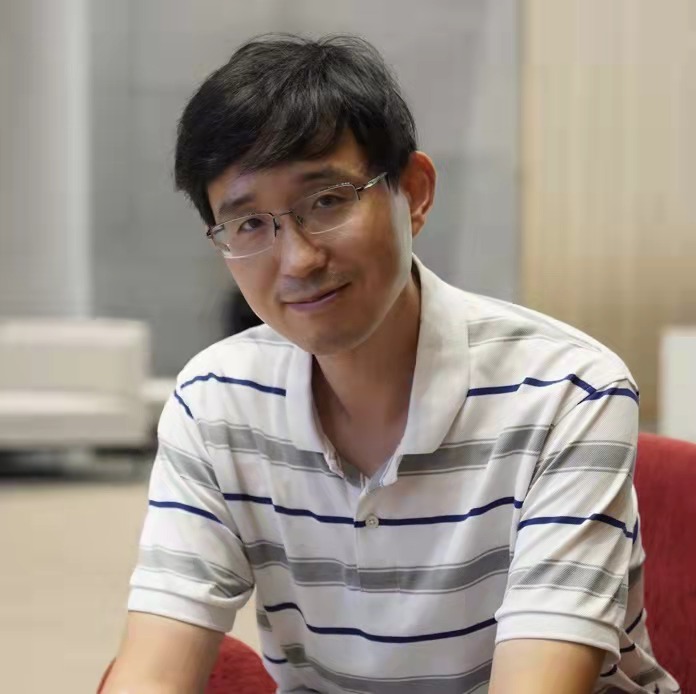
Yang You
National University of Singapore,Presidential Young Professor
Personal profile:Yang You is a Presidential Young Professor at National University of Singapore. He is on an early career track at NUS for exceptional young academic talents with great potential to excel. He received his PhD in Computer Science from UC Berkeley. His advisor is Prof. James Demmel, who was the former chair of the Computer Science Division and EECS Department. Yang You's research interests include Parallel/Distributed Algorithms, High Performance Computing, and Machine Learning. The focus of his current research is scaling up deep neural networks training on distributed systems or supercomputers. In 2017, his team broke the world record of ImageNet training speed, which was covered by the technology media like NSF, ScienceDaily, Science NewsLine, and i-programmer. In 2019, his team broke the world record of BERT training speed. The BERT training techniques have been used by many tech giants like Google, Microsoft, and NVIDIA. Yang You’s LARS and LAMB optimizers are available in industry benchmark MLPerf. He is a winner of IPDPS 2015 Best Paper Award (0.8%), ICPP 2018 Best Paper Award (0.3%) and ACM/IEEE George Michael HPC Fellowship. Yang You is a Siebel Scholar and a winner of Lotfi A. Zadeh Prize. Yang You was nominated by UC Berkeley for ACM Doctoral Dissertation Award (2 out of 81 Berkeley EECS PhD students graduated in 2020). He also made Forbes 30 Under 30 Asia list (2021) and won IEEE CS TCHPC Early Career Researchers Award for Excellence in High Performance Computing.
Topic:Colossal-AI: A Unified Deep Learning System For Large-Scale Parallel Training
Abstract(Optional):
The Transformer architecture has improved the performance of deep learning models in domains such as Computer Vision and Natural Language Processing. Together with better performance come larger model sizes. This imposes challenges to the memory wall of the current accelerator hardware such as GPU. It is never ideal to train large models such as Vision Transformer, BERT, and GPT on a single GPU or a single machine. There is an urgent demand to train models in a distributed environment. However, distributed training, especially model parallelism, often requires domain expertise in computer systems and architecture. It remains a challenge for AI researchers to implement complex distributed training solutions for their models.
In this paper, we introduce Colossal-AI, which is a unified parallel training system designed to seamlessly integrate different paradigms of parallelization techniques including data parallelism, pipeline parallelism, multiple tensor parallelism, and sequence parallelism. Colossal-AI aims to support the AI community to write distributed models in the same way as how they write models normally. This allows them to focus on developing the model architecture and separates the concerns of distributed training from the development process. The documentations can be found at this https://www.colossalai.org/ and the source code can be found at this https://github.com/hpcaitech/ColossalAI.
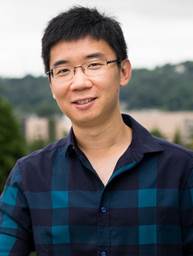
Jialin Li
National University of Singapore,Assistant Professor in the School of Computing
Personal profile:Jialin Li is an Assistant Professor in the School of Computing at the National University of Singapore. He finished his PhD at the University of Washington in 2019, advised by Dan R. K. Ports and Arvind Krishnamurthy. As part of his dissertation work, he has built practical distributed systems that offer both strong semantics and high performance, by co-designing with new-generation programmable hardware. He is the recipient of best paper awards at OSDI and NSDI. He received his B.S.E in Computer Engineering from the University of Michigan in 2012.
Topic:Co-Designing Distributed Systems with Programmable Networking Hardware
Abstract:
With the end of Dennard scaling and Moore's Law, performance improvement of general purpose processors has been stagnant for the past decade. This is in contrast to the continuous growth in network speed in data centers and telecommunication networks, and the increasing demand of modern applications. Not surprisingly, software processing on CPUs has become the performance bottleneck of many large scale distributed systems deployed in data centers.
In this talk, I will introduce a new approach to designing distributed systems in data centers that tackle the aforementioned challenge -- by co-designing distributed systems with the data center network. Specifically, my work has taken advantage of new-generation programmable switches in data centers to build novel network-level primitives with near-zero processing overhead. We then leverage these primitives to enable more efficient protocol and system designs. I will describe several systems we built that demonstrate the benefit of this approach. The first two, Network-Ordered Paxos and Eris, virtually eliminate the coordination overhead in state machine replication and fault-tolerant distributed transactions, by relying on network sequencing primitives to consistently order user requests. The third system, Pegasus, substantially improves the load balancing of a distributed storage system -- up to a 9x throughput improvement over existing solutions -- by implementing an in-network coherence directory in the switching ASICs. I will end the talk with our most recent work on accelerating permissioned blockchain systems using this co-design approach.
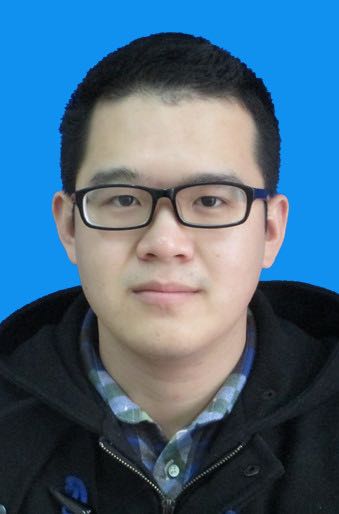
Liangfeng Cheng,Ph.D student
Personal profile:Liangfeng Cheng is now a Ph.D student in Huazhong University of Science and Technology (HUST) advised by Prof. Yuchong Hu. He received the B.Eng. degree in Huazhong University of Science and Technology (HUST) in 2017. Then, he took a successive postgraduate and doctoral program in 2019. His research mainly focuses on computer architecture and cloud storage including erasure coding, in-memory key-value stores, data deduplication, etc.
Topic:LogECMem: Coupling Erasure-Coded In-Memory Key-Value Stores with Parity Logging
Abstract:
In-memory key-value stores are often used to speed up Big Data workloads on modern HPC clusters. To maintain their high availability, erasure coding has been recently adopted as a low-cost redundancy scheme instead of replication. Existing erasure-coded update schemes, however, have either low performance or high memory overhead. In this paper, we propose a novel parity logging-based architecture, HybridPL, which creates a hybrid of in-place update (for data and XOR parity chunks) and log-based update (for the remaining parity chunks), so as to balance the update performance and memory cost, while maintaining efficient single-failure repairs. We realize HybridPL as an in-memory key-value store called LogECMem, and further design efficient repair schemes for multiple failures. We prototype LogECMem and conduct experiments on different workloads. We show that LogECMem achieves better update performance over existing erasure-coded update schemes with low memory overhead, while maintaining high basic I/O and repair performance
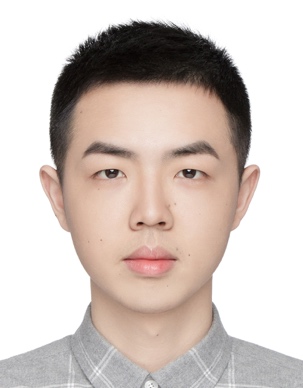
Yu Huang,Ph.D. student
Personal profile:Yu Huang is a CS Ph.D. student at Huazhong University of Science and Technology, supervised by Prof. Xiaofei Liao and Long Zheng. His research interests focus on computer architecture and systems, especially processing-in-memory and graph processing. He received the BS degree from the Huazhong University of Science and Technology in 2016.
Topic:Accelerating Graph Convolutional Networks Using Crossbar-based Processing-In-Memory Architectures
Abstract:
Graph convolutional networks (GCNs) are promising to enable machine learning on graphs. GCNs exhibit mixed computational kernels, involving regular neural-network-like computing and irregular graph-analytics-like processing. Existing GCN accelerators follow a divide-and-conquer philosophy to architect two separate types of hardware to accelerate these two types of GCN kernels, respectively. This hybrid architecture improves intra-kernel efficiency but considers little inter-kernel interactions in a holistic view for improving overall efficiency. In this work, we present a new GCN accelerator, ReFlip, with three key innovations in terms of architecture design, algorithm mappings, and practical implementations. First, ReFlip leverages PIM-featured crossbar architectures to build a unified architecture for supporting the two types of GCN kernels simultaneously. Second, ReFlip adopts novel algorithm mappings that can maximize potential performance gains reaped from the unified architecture by exploiting the massive crossbar-structured parallelism. Third, ReFlip assembles software/hardware co-optimizations to process real-world graphs efficiently. Results show that ReFlip can significantly outperform the state-of-the-art CPU, GPU, and accelerator solutions in terms of both performance and energy efficiency.
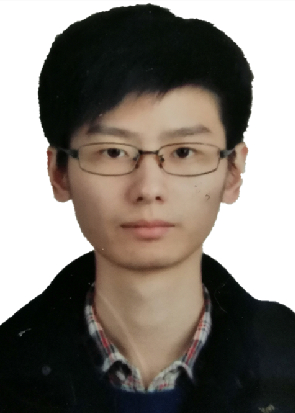
Zhong Yang,Ph.D student
Personal profile:Yang Zhong is a Ph.D student in the School of Computer Science and Technology, Huazhong University of Science and Technology. His research interests are data management and data science with a focus on developing novel algorithm for set/string similarity search. He develops adaptive algorithms for top-k set similarity joins and efficient algorithms for string similarity search under edit distance. He received his Master degree in Nanyang Technological University.
Topic:Efficient Algorithms for Set/String Similarity Search
Abstract:
Set/String similarity search is one of the essential operating in data processing, it has a broad range of applications including data cleaning, near-duplicate object detection and data integration. The top-k set similarity join is a variant of the threshold-based set similarity join to avoid the problem of setting an appropriate threshold before-hand. The state-of-the-art solution for top-k set similarity join disregards the effect of the so-called step size, which is the number of elements accessed in each iteration of the algorithm. We propose an adaptive step size algorithm that is capable of automatically adjusting the step size, thus taking advantage of the benefits of large step sizes as well as reducing redundant computations. We also study the threshold string similarity search problem under edit distance. Previous proposals for the problem suffer from huge space consumption issue when achieving only an acceptable efficiency, especially for long strings. To eliminate the issue, we propose a simple and small index, called minIL. We first adopt a minhash family to capture pivot characters and to construct sketch representations for strings, and then develop a succinct multi-level inverted index to search the sketches with low space cost and high efficiency.